Empowering healthcare with quality data and retrieval indexing through GenAI
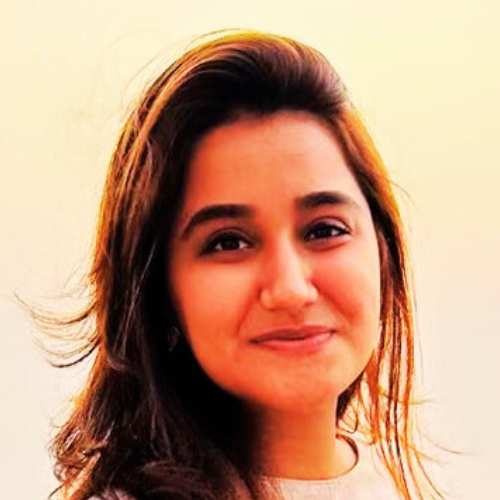
Ruchi Gohil
Senior Healthcare BA, Citius Healthcare Consulting
Sep - 30
Article
Incorporating Generative AI (GenAI) into healthcare opens up exciting possibilities, but its true potential can only be unlocked with a solid data foundation. By focusing on critical initial steps—such as cleaning and indexing data for efficient retrieval—healthcare organizations can set the stage for GenAI to thrive. This article will explore the practical approaches for achieving impeccable data quality and efficient indexing to fully harness Gen AI’s capabilities in transforming healthcare.
Ensuring data quality for better healthcare outcomes
In healthcare, precise data is the linchpin for effective decision-making, patient outcomes, and cost management. Even the most advanced AI models, like GenAI, can falter without high-quality data. Here are the key steps to ensure data quality:
- Data cleaning: Eliminating inaccuracies and inconsistencies from data is crucial to maintaining high data quality. Techniques like data normalization and validation rules are key in enhancing data integrity. For instance, standardizing medical terminologies and coding systems (e.g., ICD, CPT) across datasets ensures uniformity, which is critical for GenAI models to interpret and analyze data accurately.
- Data enrichment: Supplementing raw data with additional contextual information can enhance its value and usability. In healthcare, this might involve integrating clinical data with socioeconomic determinants of health, patient-reported outcomes, and wearable device data. Enriched data provides GenAI with a more comprehensive view of the patient, enabling more personalized care insights.
Indexing for efficient retrieval in GenAI applications
Retrieval indexing is essential for quick data access and retrieval, particularly in emergency settings. Here’s how healthcare organizations can optimize data indexing:
- Structured data storage: Utilizing database management systems (DBMS) that support fast indexing and querying, such as SQL databases for structured data. Indexing techniques like B-trees or hash indexing can optimize data retrieval speeds, enabling GenAI applications to process large datasets quickly and effectively.
- Metadata management: Comprehensive metadata repositories are crucial for cataloging data sources, data types, and relationships. This facilitates faster identification and retrieval of relevant information, empowering GenAI applications to provide actionable insights in real time.
- Role-based access controls: Implementing role-based access control (RBAC) systems prevents unauthorized access and ensures data privacy. This strategy aids in managing who can access, modify, or add data, ensuring GenAI applications comply with regulations like HIPAA and GDPR.
Optimizing data for implementing GenAI solutions in healthcare
To further improve data quality and indexing capabilities, consider these practical steps:
- Automated data ingestion and processing: Using ETL (Extract, Transform, Load) tools can automate data extraction from various sources, transform it into standardized formats, and load it into unified databases. This process ensures high-quality, standardized data, which in turn enhances the efficiency of Gen AI models.
- Data classification and tagging: Employing machine learning algorithms can classify and tag unstructured data (e.g., medical notes and imaging reports) into structured formats. Techniques like NLP (Natural Language Processing) can parse text data into categorizable entities, which is vital for Generative AI to analyze unstructured healthcare data effectively.
- AI-driven indexing: Leveraging AI to create dynamic indices that adapt to data usage patterns can optimize data retrieval for GenAI models. By predicting the most frequently accessed data, AI can adjust indexing strategies to improve performance and accessibility.
Enhancing clinical decision support with GenAI
For clinical decision support systems, the primary goal is to provide actionable insights based on high-quality data. Here’s how:
- Predictive analytics: GenAI can develop predictive models using historical patient data to forecast outcomes and assist in treatment planning. For example, Closed Loop and Althea have demonstrated how AI can predict preventable conditions by analyzing comprehensive health data.
- Interoperability standards: Ensuring compatibility with existing healthcare standards such as HL7 and FHIR is crucial for GenAI implementations. Interoperable systems allow for seamless data exchange and integration with disparate healthcare applications.
Ensuring data privacy and security in GenAI implementation
As healthcare organizations embrace GenAI, ensuring data privacy and security remains a top priority. Effective measures need to be in place to protect sensitive health information:
Case studies highlighting the importance of data quality
- ECG digitalization: Implementing digital time-series data for ECG, coupled with machine learning for heart failure prediction, significantly improved prediction accuracy compared to traditional analog scans. This highlights the importance of error-free, standardized data in AI-driven healthcare innovations.
- Data flow diagrams in ICUs: Aggregating data from various patient monitoring devices into a single research database, controlled by role-based access, ensures data privacy while enabling comprehensive analytics. This approach demonstrates how data management supports both GenAI and patient care.
Conclusion
Generative AI has transformative potential in healthcare, but its efficacy is contingent upon the quality of the underlying data and the efficiency of indexing mechanisms. By adopting rigorous data cleaning, enrichment, structured storage, and AI-driven indexing, healthcare organizations can unlock the full potential of GenAI, driving significant improvements in patient care, operational efficiency, and cost management. As business analysts, we play a significant role in guiding healthcare organizations toward these foundational practices, ensuring their readiness to capitalize on the transformative capabilities of GenAI.